Table Of Content
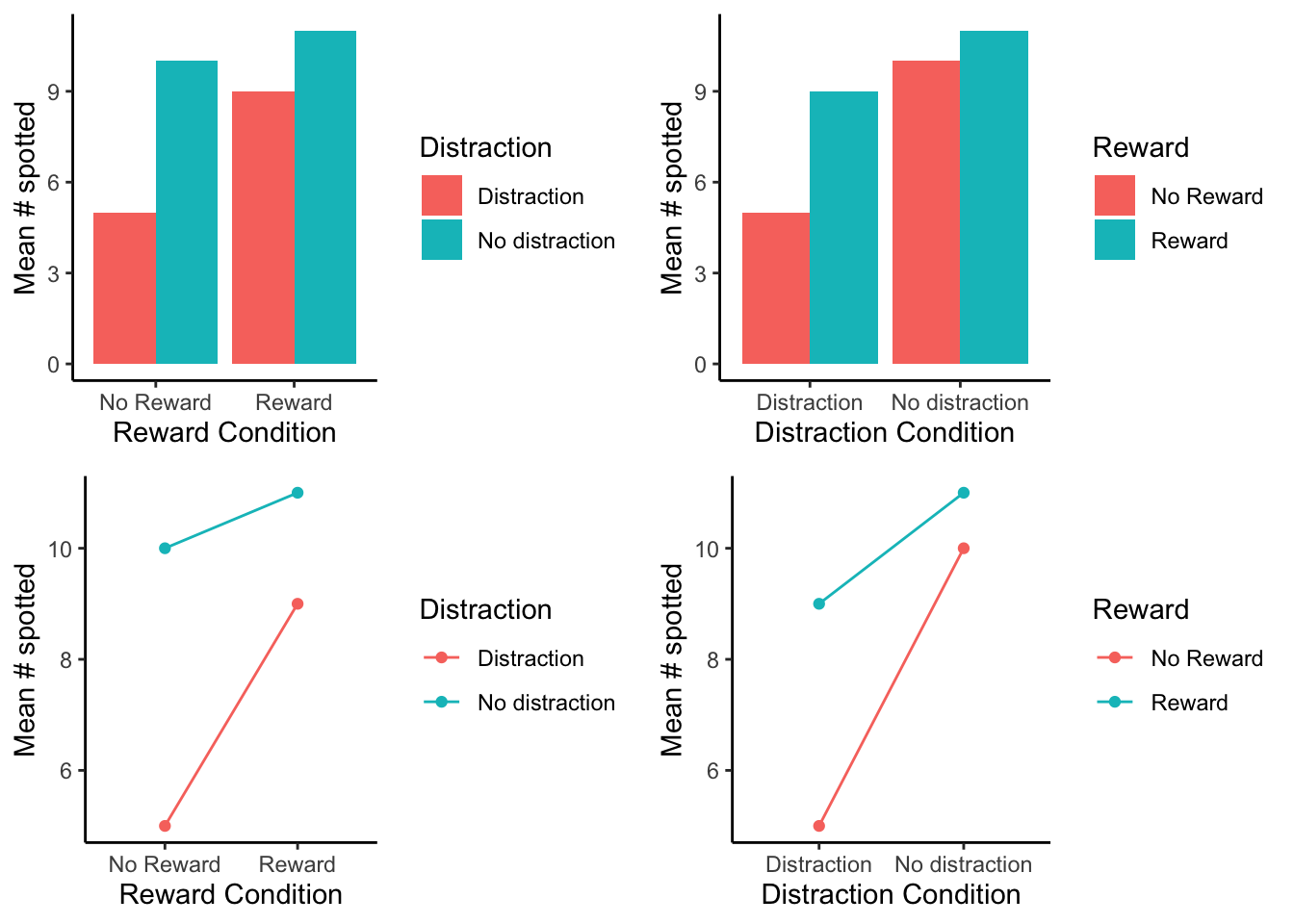
When you look for the differences, it feels like you are doing something we would call “paying attention”. If you pay attention to the clock tower, you will see that the hands on the clock are different. You look at two pictures side-by-side in Figure 9.3, and then you locate as many differences as you can find.
How do we get temporal and dispersion derivatives on SPM? - ResearchGate
How do we get temporal and dispersion derivatives on SPM?.
Posted: Thu, 07 May 2015 07:00:00 GMT [source]
Google Sheets: How to Use SEARCH with Multiple Values
Another important use of complex correlational research is to explore possible causal relationships among variables. This might seem surprising given that “correlation does not imply causation”. It is true that correlational research cannot unambiguously establish that one variable causes another. Complex correlational research, however, can often be used to rule out other plausible interpretations. Onwards, the minus (−) and plus (+) signs will indicate whether the factor is run at a low or high level, respectively.
2.4 Distraction effect
We can look at this two ways, and either way shows the presence of the very same interaction. First, does the effect of being tired depend on the levels of the time since last meal? Look first at the effect of being tired only for the “1 hour condition”.
2.5 Manipulating the Distraction effect
So, we did find that the difference (in the distraction effect) between the differences (the two measures of the distraction effect between the reward conditions) were different. When you start to write down explanations of what interactions are, you find out why they come across as complicated. We’ll leave our definition of interaction like this for now. Don’t worry, we’ll go through lots of examples to help firm up this concept for you. Certain research questions may require understanding know how each factor may independently impact a dependent variable. For example, observed changes in worker productivity scores due to salary are separated from those due to skill level, to help determine the main effects for each.
CEQUEL: Combo therapy a win in bipolar depression - MDedge
CEQUEL: Combo therapy a win in bipolar depression.
Posted: Tue, 25 Oct 2016 07:00:00 GMT [source]
Because you ran the design with more than one IV, you have the opportunity to ask these kinds of extra questions. Maybe there is a special drug that helps you ignore distracting things. People taking this drug should be less distracted, and if they took this drug while completing our task, they should have a smaller distraction effect compared to people not taking the drug.
While this algorithm is fairly straightforward, it is also quite tedious and is limited to 2n factorial designs. Thus, modern technology has allowed for this analysis to be done using statistical software programs through regression. Suppose you have two variables \(A\) and \(B\) and each have two levels a1, a2 and b1, b2.
Consider some research by Paul Piff and his colleagues, who hypothesized that being lower in socioeconomic status (SES) causes people to be more generous [PKCote+10]. They measured their participants’ SES and had them play the “dictator game.” They told participants that each would be paired with another participant in a different room. (In reality, there was no other participant.) Then they gave each participant 10 points (which could later be converted to money) to split with the “partner” in whatever way he or she decided.
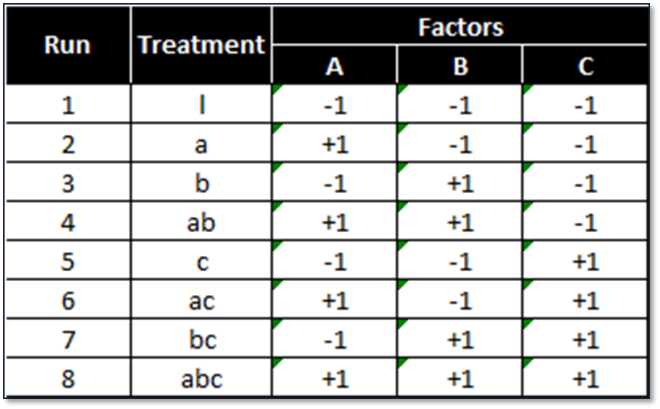
2 Purpose of Factorial Designs
Notice, however, that the values are all relative to one another. So while the largest main total effect value in one set of experiments may have a value of 128, another experiment may have its largest main total effect value be 43. In the previous section, we looked at a qualitative approach to determining the effects of different factors using factorial design. Now we are going to shift gears and look at factorial design in a quantitative approach in order to determine how much influence the factors in an experiment have on the outcome. A null outcome situation is when the outcome of your experiment is the same regardless of how the levels within your experiment were combined.
As with simple designs with only one independent variable, factorial designs have the same basic empirical question. Did manipulation of the independent variables cause changes in the dependent variables? However, 2x2 designs have more than one manipulation, so there is more than one way that the dependent variable can change. So, we end up asking the basic empirical question more than once. Factorial designs require the experimenter to manipulate at least two independent variables.
For example, measures of warmth, gregariousness, activity level, and positive emotions tend to be highly correlated with each other and are interpreted as representing the construct of extraversion. As a final example, researchers Peter Rentfrow and Samuel Gosling asked more than 1,700 university students to rate how much they liked 14 different popular genres of music [RG03]. They then submitted these 14 variables to a factor analysis, which identified four distinct factors. Most complex correlational research, however, does not fit neatly into a factorial design.
The researchers dealt with these potential third variables, however, by measuring them and including them in their statistical analyses. They found that neither religiosity nor ethnicity was correlated with generosity and were therefore able to rule them out as third variables. This does not prove that SES causes greater generosity because there could still be other third variables that the researchers did not measure. But by ruling out some of the most plausible third variables, the researchers made a stronger case for SES as the cause of the greater generosity.
Then we will see how much additional variation they explain beyond the group means for reward and distraction. To do this we treat each score as the condition mean for that score. Then we subtract the mean for the distraction group, and the mean for the reward group, and then we add the grand mean. This gives us the unique variation that is due to the interaction. We could also say that we are subtracting each condition mean from the grand mean, and then adding back in the distraction mean and the reward mean, that would amount to the same thing, and perhaps make more sense.
This will be true no matter whether they wear a hat or not, and no matter how tall the hat is. In other words, the effect of wearing a shoe does not depend on wearing a hat. More formally, this means that the shoe and hat independent variables do not interact. It would mean that the effect of wearing a shoe on height would depend on wearing a hat. But in some other imaginary universe, it could mean, for example, that wearing a shoe adds 1 to your height when you do not wear a hat, but adds more than 1 inch (or less than 1 inch) when you do wear a hat. This thought experiment will be our entry point into discussing interactions.
The overall means, averaging over subjects are in the bottom green row. This is the difference between the AC column (average of subject scores in the no-distraction condition) and the BD column (average of the subject scores in the distraction condition). The interaction effects situation is the last outcome that can be detected using factorial design.
No comments:
Post a Comment